Optimizing schedules is hard. Custom algorithms that leverage ML and Mathematical Optimization can help make it easy.
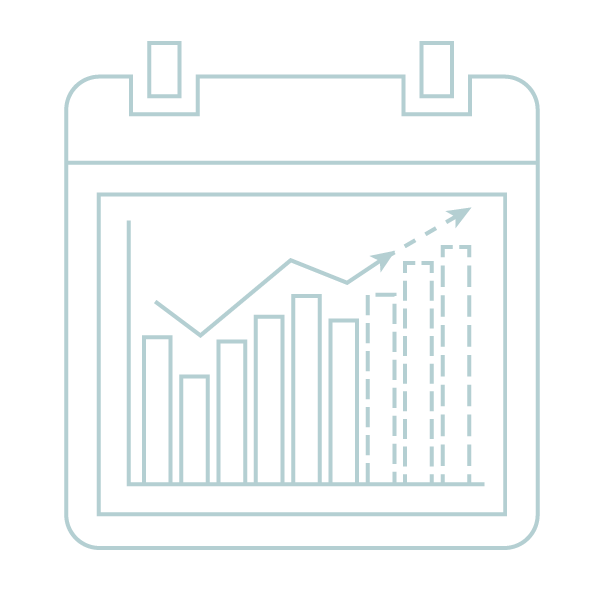
Blended ML & Optimization Should Solve Scheduling Problems
Staffing and scheduling optimization are crucial for many industries, significantly when the exact timing of high-volume activity can change based on complex factors. Augmenting the workforce and assets too early means diverting profit to unnecessary costs while waiting until high-volume demand is already underway, risking operating below capacity and decreasing customer satisfaction (e.g., if there are long wait times).
Machine learning can be deployed to forecast operational demand and predict event duration. Mathematical optimization can augment these ML inputs to recommend a schedule that optimizes against your unique scheduling objectives.
Mosaic Data Science has deep expertise in developing custom algorithms to solve highly complex scheduling problems.
Featured Scheduling Optimization Tools
Why Airlines Need to Look at Holistic Machine Learning & Optimization Solutions to Improve Scheduling
Making Time for Scheduling Optimization: Q&A With Evan Lynch, Principal Data Scientist
We scheduled some time for this Q&A with Principal Data Scientist Evan Lynch, where we explored the many cross-industry challenges that scheduling optimization aims to solve, and the robust scheduling ...
Surgeon OR Scheduling Optimization
Mosaic helped a leading hospital optimize the scheduling of elective surgery and created a better daily rhythm for surgeons.
Integrated Machine Learning and Mathematical Optimization
For the past several years, ML has exploded in popularity, while the excitement for MO has mostly plateaued. Why this has occurred is very much up for debate. One might ...
Workforce Planning Prediction
Optimizing seasonal staffing and resourcing is a key challenge for many industries, especially when the exact timing of high-volume activity can change based on complex factors.
Scheduling Problems Persist Despite Advancements in ML
- An assignment problem, assigning resources to entities that require them,
- A schedule is a sequence, an assignment problem that involves order,
- A schedule almost always has a start time and duration.
Mosaic built a custom AI scheduling tool to optimize surgery scheduling for a hospital system.
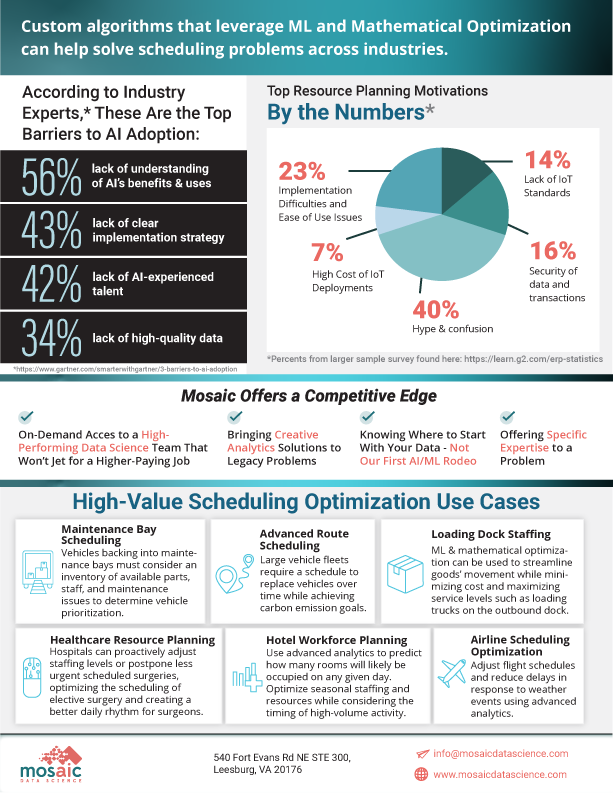
Download our Scheduling Optimization Tools Sheet
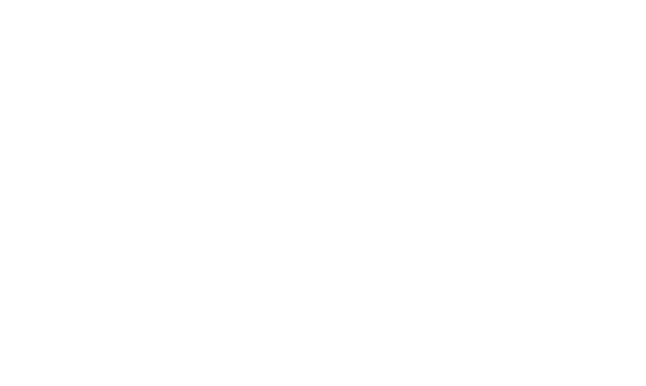