Summary
We helped one of the largest Children’s Healthcare Network more accurately forecast patient volume across their hospitals.
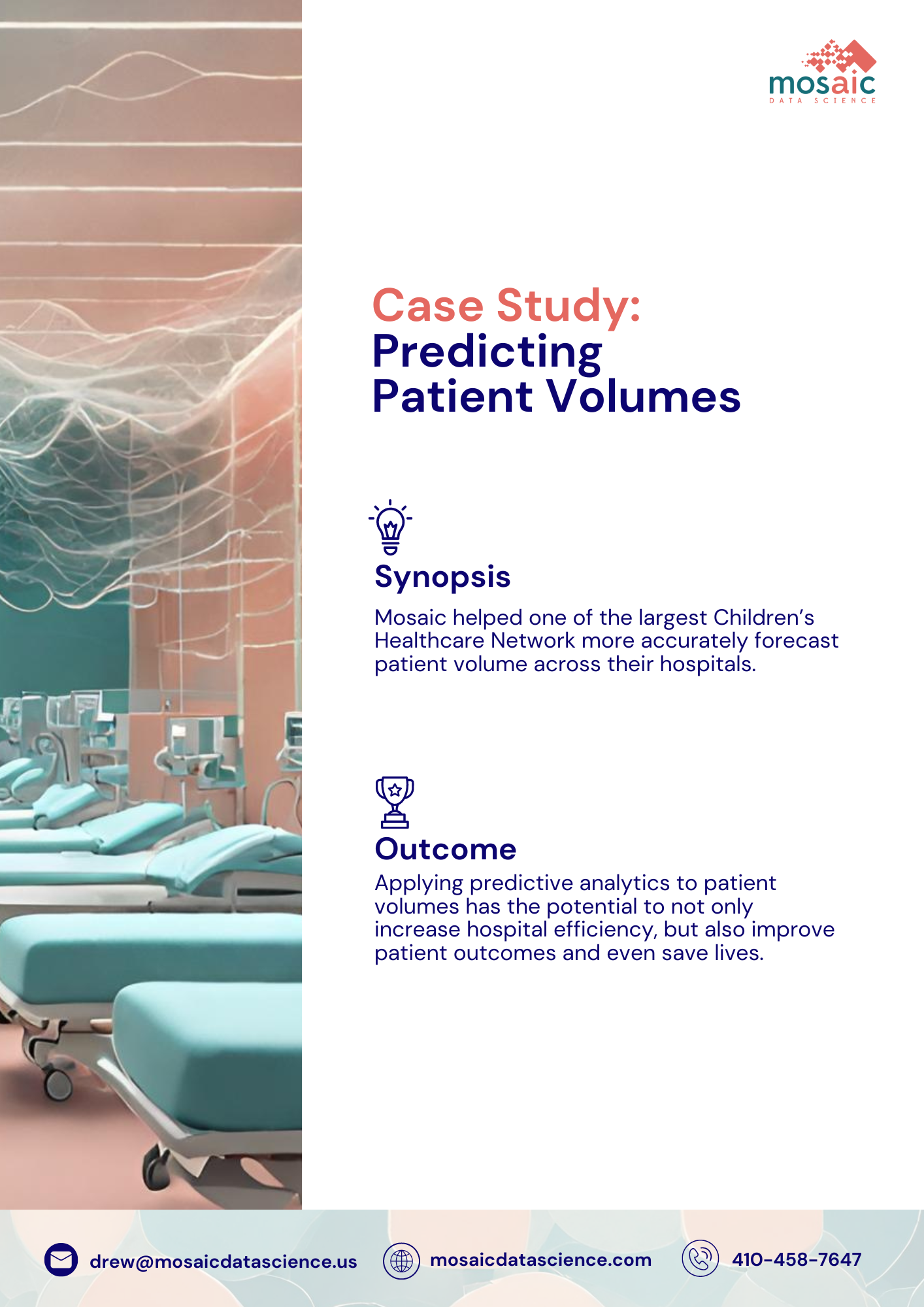
Take Our Content to Go
Background
A leading not-for-profit children’s hospital system had seen an increase in the last two years in the number and duration of children’s Intensive Care Unit (ICU) saturation events. A saturation event occurs when the bed capacity and/or staffing levels in the ICU are not sufficient to cover patient volumes. During these events, patient levels must be controlled by, for example, raising thresholds for ICU admission or redirecting incoming Emergency Department (ED) patients to other hospitals in the network that may not be as well-equipped to provide best possible treatment. Obviously, these reactive workarounds are not ideal for the patient or hospital. With more foresight, however, the hospital can proactively adjust staffing levels or postpone less urgent scheduled surgeries that can involve ICU recovery time in order to open up more ICU capacity and avoid a saturation event. The healthcare organization believed predictive analytics could help them lessen the frequency of saturation events or shorten their duration so they could better serve critical ICU and ED patients.
Mosaic Data Science, a top healthcare machine learning consulting firm, was engaged based on its depth of expertise in predictive analytics development and deployment. This was the first time the hospital system would apply predictive analysis and data science to its internal hospital administration. Mosaic worked in close collaboration with the healthcare organization to define the predicting patient volumes problem and develop objectives at the beginning of the project. After these discussions, the two organizations agreed that the best plan of action was to develop a suite of predictive models that would better predict ED patient levels, which are the greatest source of variation for ICU admission rates. The outputs from these models would allow the hospital system to implement strategies to proactively mitigate against saturation events.
Predicting Patient Volumes | Model Design
To predict ICU admission rates, Mosaic’s data science consultants needed to both accurately predict ED visits and accurately measure ICU patient volumes. The healthcare provider had 4 years of historical data on patient volumes, staffing schedules, and known saturation events available that could serve as a training dataset for a predictive model. The model would need to predict the weekly inflow of ED patients and the corresponding expected ICU admission rates out to a 12-week time horizon.
Mosaic tested 3 different time series forecasting techniques: ARIMA, seasonal exponential smoothing (Holt-Winters), and time series regression. Over 25 different model formulations were tested during the model training phase. Mean absolute error (MAE) and mean absolute percent error (MAPE) at different time horizons were used as the primary evaluation metrics. These were calculated by running out-of-sample validation data through the time series models to test model accuracy at predicting future ED visits based on historical data. The following graphics show the error between actual vs. predicted ED visits.
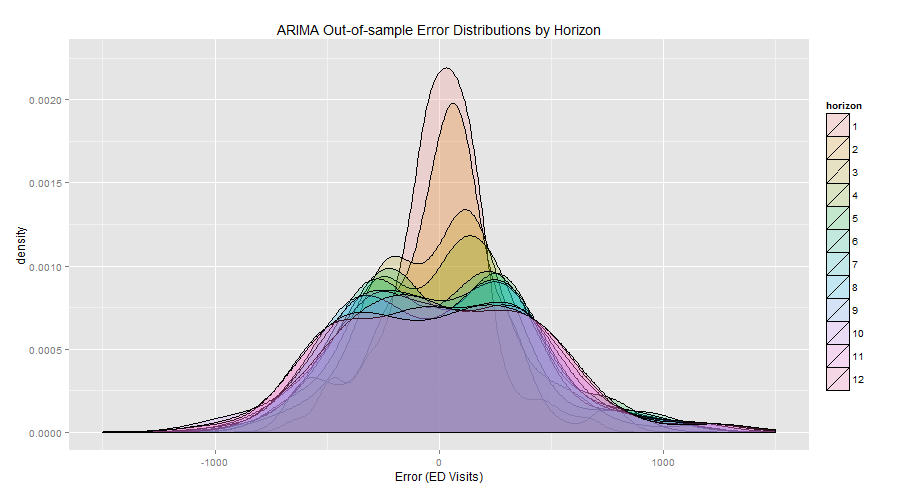
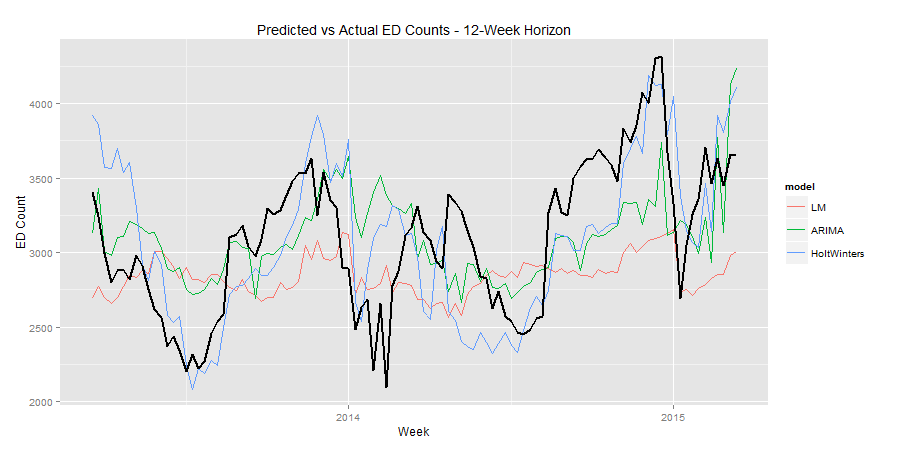
An ensemble model was applied to help smooth out variability in errors by combining results from the 3 independent models. This forecasting exercise confirmed an insight: seasonality, or time of year, had a very large impact on ED visits. With this knowledge, the organization can dig deeper into the data to determine which months are busiest and begin to implement strategies to reduce saturation events during the busiest times.
Model Deployment
After Mosaic implemented and validated these time series forecasts, the hospital system wanted to determine what factors had the most significant impacts on ED visits. Mosaic segmented forecasts by 2 hospital locations, patient diagnosis groups (e.g., asthma, vomiting, bronchiolitis), patient acuity at admission, and day and time of patient arrival. The same ensembling method used for the overall forecasts proved quite effective at generating sub-forecasts for each patient segment. The resulting sub-forecasts allowed the healthcare provider to understand which patient groups were driving the greatest variability in ED arrival rates and how much of this variability within each segment could be accurately forecast.
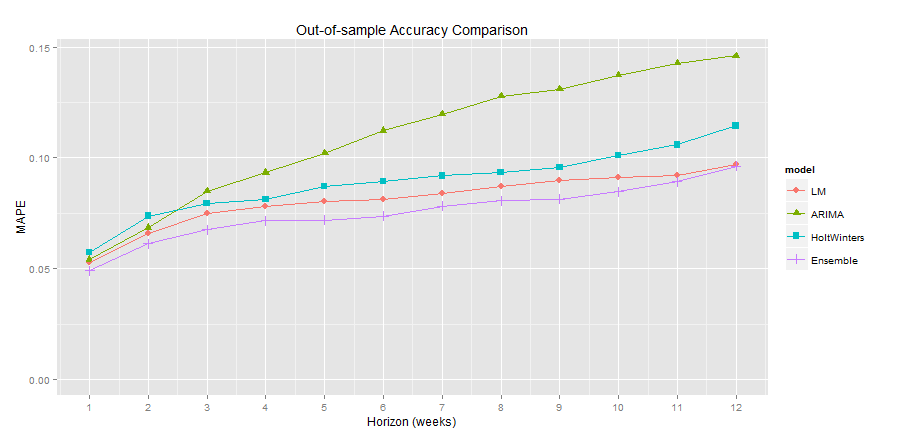
Results
Mosaic Data Science designed and developed forecasting approaches that produced accurate ED patient inflow forecasts up to 12 weeks in advance. Figure 3 shows that Mosaic was able to achieve a MAPE for the ensemble model of under 10% at a look-ahead horizon up to 12 weeks into the future. A hospital that is able to accurately predict patient volumes, especially in such critical departments as the ED and ICU, can enact mitigation strategies to make sure the highest quality care is provided to patients under all sorts of operating conditions. Applying predictive analytics to patient volumes has the potential to not only increase hospital efficiency, but also improve patient outcomes and even save lives.