Summary
Using AI to analyze sensor data, Mosaic helped a renewable energy producer optimize furnace temperature to improve wood pellet production efficiency by reducing slag production and pressure buildup and providing control room operators with easy-to-use recommendations for setting furnace controls.
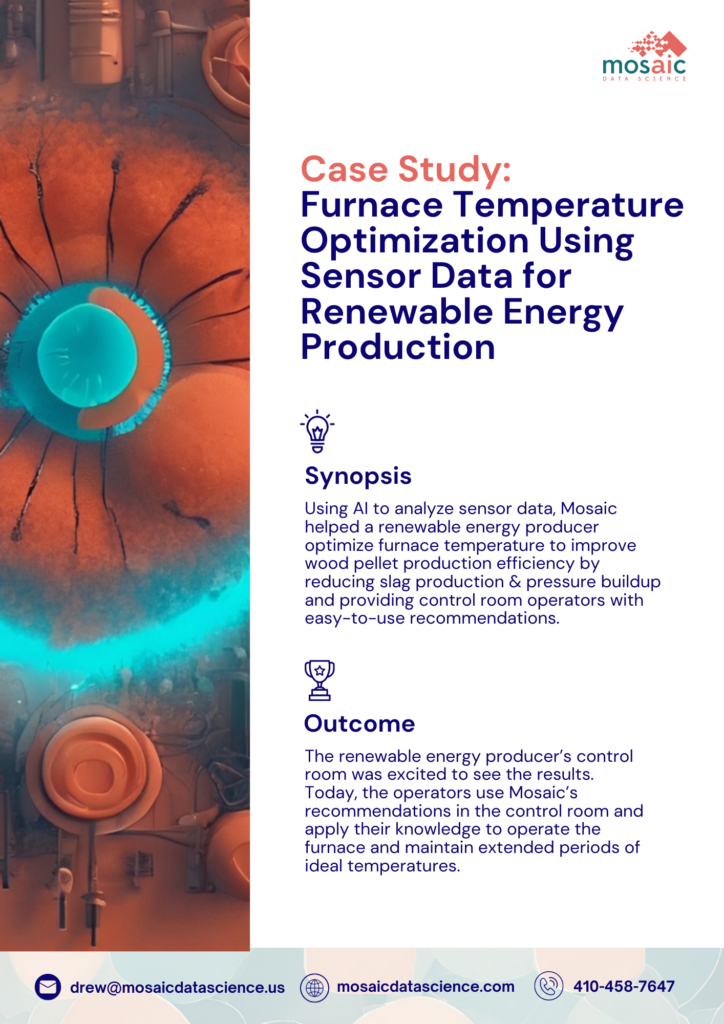
Take Our Content to Go
The Need for an AI in Manufacturing Processes
Manufacturing is a complex process that involves many moving parts. Producers need to be able to make quick and accurate decisions to succeed. AI-Powered Manufacturing Optimization can help firms to identify patterns and trends that would be difficult or impossible to spot with the naked eye.
By using AI to analyze sensor data and other internal data assets, operators can predict mechanical failures, identify bottlenecks in the production process, optimize inventory levels, improve product quality, and reduce operating costs. Tailored optimization and AI tools can help any manufacturing company minimize downtime and maximize efficiency.
Introduction
One of the key processes in the production of wood pellets is the removal of moisture, which makes up more than 50% of the raw input wood fiber. The manufacturer sources a wide variety of raw wood fiber, including wood chips, whole trees, sawdust, and bark. The goal of the drying process is to remove excess moisture from the raw input with the aim of hitting a consistent outlet moisture target.
The dryer drum is a single-pass dryer with a motor that rotates the drum around its center axis. Green (raw) wood chips are pushed through the drum from one end, and dried chips come out the other end before being transferred to downstream processes (e.g., sizing, pellet production, etc.).
For the purposes of this case study, Mosaic’s capabilities were initially centered around the management of the furnace that supplies constant heat to the drying process. Our team partnered with one of the world’s largest producers of renewable energy to kickstart its advanced analytics program by optimizing the process for determining the appropriate starting point temperature for the furnace. The customer operates ten manufacturing facilities across the U.S. that produce wood pellets, a renewable alternative to coal.
As the renewable energy producer ramped up their analytics capabilities, they engaged Mosaic for flexible access to help run a pair of use cases that focused on operational improvements at their pellet production plants. Mosaic’s hands-on team helped the renewable energy producer make sense of their large amounts of data and match the appropriate analytics technique to their use case, ultimately creating a custom and user-friendly recommender tool.
Identifying the Problem for AI-Powered Manufacturing Optimization Improvements
When removing moisture from raw wood, the furnace is a massive piece of equipment where a fire, fueled by bark, is kept constantly burning. The heat produced by the furnace is used to dry the wood chips in the dryer and can reach temperatures as high as 1,800 degrees Fahrenheit. The furnace process receives and combusts biomass fuel (bark) to produce hot air that is supplied as the primary heating source for the dryer. The fuel is fed into the lower chamber of the furnace, where a series of moving and fixed grates help push fuel along throughout the combustion process. The grates push the burning fuel down an incline to separate the ash and allow more fuel.
Operators in the control room can control several different furnace settings, which work in tandem to manage the fire to a specific temperature range. These settings are:
- Fuel feed rate: Rate at which new fuel is fed into the furnace.
- Grate speeds: Rate at which the moving grates push fuel along.
- Primary air fan speed: Rate at which oxygen is supplied to the fire.
- Secondary air damper position: A second oxygen source above the fire, controlled by a damper.
The primary challenge is that control room operators struggle to understand the relationship between these control settings and how they need to be set to maintain a specific temperature. While one may think the goal of operators is simply to maximize the furnace temperature, the intent is most often to optimize the control settings to hit a specific temperature range. This is due to safety and equipment maintenance considerations.
When the furnace is operated at above-normal temperatures, the customer risks the production of melted ash, also known as slag, which interferes with the combustion process and damages the furnace and conveyors. Operators are also monitoring the pressure buildup at higher temperatures. If the pressure gets too high, this poses a safety issue for those at the production sites. Control room operators needed an easy-to-use tool that could recommend the best starting point for each setting as they ramped up or down the furnace temperature.
AI-Powered Manufacturing Optimization Development
The renewable energy producer had been collecting data from hundreds of different sensors throughout their production process. These sensors continuously collect data relating to the status and performance of the furnace, including temperature readings at different points within the furnace, speeds of different system components, set points of system controls, moisture content readings, and more.
The data collected was stored in an SQL database that was refreshed multiple times daily. While hundreds of sensors were used, we narrowed our focus to the sensors the control room operators used to manage (control settings mentioned above) and gauge the furnace’s performance (temperature). By isolating these sensor readings, Mosaic was able to show how furnace operators have run the furnace historically and highlight the various ways to achieve a desired target temperature for extended periods of time.
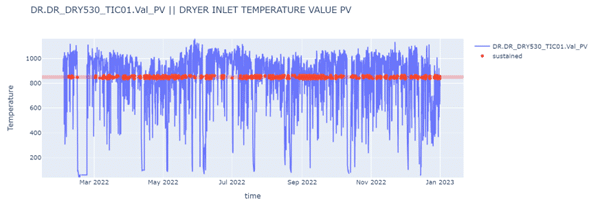
AI-Powered Manufacturing Optimization Figure 1: Furnace Control & Temperature Sensor Readings
From our exploratory analysis, Mosaic dove in to answer the following questions:
- How frequently does the furnace maintain a consistent temperature?
- What furnace settings are used most often during periods of sustained temperature?
Mosaic began by measuring how frequently the control room was able to maintain an array of temperature targets (e.g., 1700-1725, 1725-1750, etc.) for a period of at least three hours. Once Mosaic was able to find these periods of sustained temperature, we looked at the distribution of the settings.
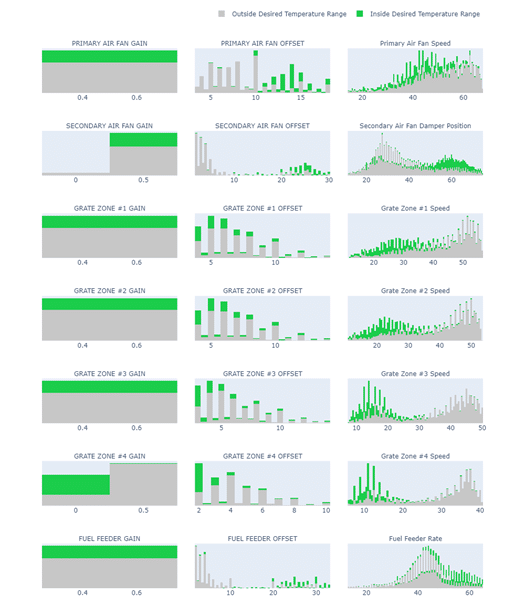
AI-Powered Manufacturing Optimization Figure 2: Temperature Target Frequency Distribution
Mosaic began by measuring how frequently the control room was able to maintain an array of temperature targets (e.g., 1700-1725, 1725-1750, etc.) for a period of at least three hours. Once Mosaic was able to find these periods of sustained temperature, we looked at the distribution of the settings.
Finally, Mosaic used the median of these distributions to provide control room operators with the best-known starting point for each setting for future adjustments. While a good starting point, factors such as input fuel moisture, ambient temperature, and others play a big role in what the exact setting should be. While further investigation and modeling are required to tease out these more complex relationships, the control room was provided with the median and a 95% confidence interval at each temperature target as a first-pass solution. This provided the control room with a reasonable range of possibilities based on what was achieved historically.
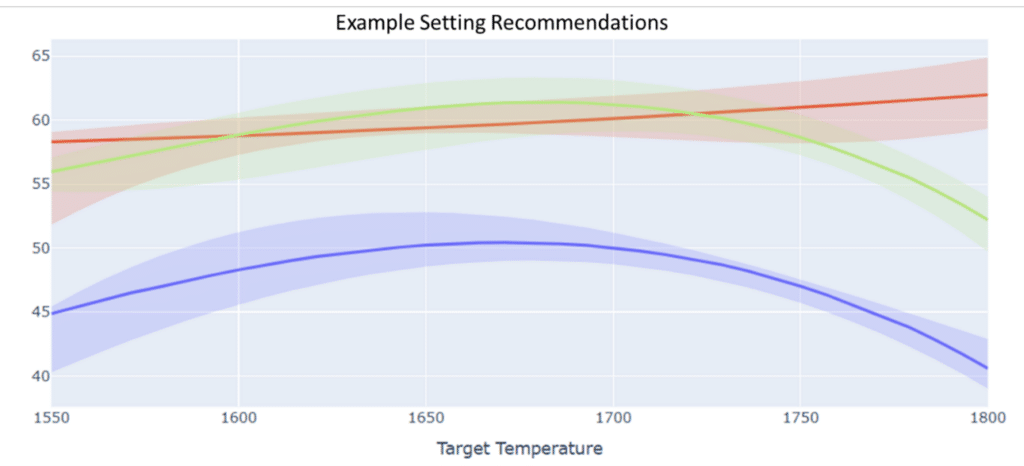
AI-Powered Manufacturing Optimization Figure 3: Example Setting Recommendations
Figure 3 is an example of recommendations for furnace operators, where we have the target temperature on the x-axis and the recommended setting value on the y-axis. The solid bold line is the recommendations, and the shaded area is the 95% confidence interval that shows the range of possible starting points.
AI-Powered Manufacturing Optimization Results
Mosaic provided HTML plots of the recommended settings so the control room could begin implementing these changes immediately. The solution was easy to understand, highlighted how the furnace operates, and was inexpensive compared to a longer model development process and full-scale web app deployment.
The renewable energy producer’s control room was excited to see the results, as the distribution of many settings across temperatures was consistent with the operational understanding of the system. Additionally, a few recommendations were surprising to the team and prompted interesting discussions about the theoretical design of the system.
AI-Powered Manufacturing Optimization Conclusion
Sensor intelligence enables more accurate decisions, empowering organizations to identify new opportunities, increase sales, improve customer experience, and optimize operational performance. Rather than replace human operators, custom-built AI & ML extract hidden information from sensor data.
Mosaic is no stranger to working with massive pools of data to build and deploy actionable solutions for our customers. Our collaborative, problem-focused, seasoned, and custom approach to advanced sensor data analytics applications made us an ideal partner to help the renewable energy producer leverage its sensor data to make the wood pellet production process more efficient. The control room began implementing Mosaic’s recommendations immediately and began seeing more consistent temperatures in the furnace. Today, the operators have the recommendations in the control room and use their knowledge in conjunction with our recommendations to operate the furnace and maintain extended periods of ideal temperatures. By turning to AI-Powered Manufacturing Optimization, the company can feel assured they are maximizing their data to streamline operations.