AI Blog Series | Post #3 in our blog series examining how leading athletic clothing retailers can place the right products at the right store at the right time using data science.
Why Predictive Inventory Planning is a great candidate for AI-driven modeling
Retailers and athletic product manufacturers continuously strive to balance demand-supply constraints and the need to be profitable. Today, automation and insights are key levers in the process of keeping the right amount of product in stock and planning for future demand. According to a National Retail Federation study, firms who deploy predictive inventory planning typically see a decrease in inventory level up to 30-50%, increased service levels up to 99% and spend up to 60% less time planning and replenishing inventory.
Firms can harness the potential of analytics in minimizing the risk of stock-outs, maximizing inventory returns and increasing brand loyalty. Data science techniques like artificial intelligence and machine learning modeling can help companies comprehensively plan their inventory based on predicted demand while accounting for other statistically significant factors.
AI consulting firms such as Mosaic Data Science would typically leverage modeling techniques such as time-series forecasting, classification, regression, supervised, and unsupervised learning to help firms, online or in-store, place the right product at the right store at the right time.
Predictive Inventory Planning | Outdoor Retailer Case Study | Automation & Accuracy
A leading outdoor lifestyle retailer sought Mosaic out to help design and develop predictive machine learning models for their merchandising teams to use in making buying decisions for size profiles in their apparel and footwear lines. Previously, all decisions were made manually based on historical sales data, and the customer data science team believed that automating much of the process, integrating new data sources, and running predictive analytics on these data streams would help the merchandising team make better decisions in a shorter timeframe.
Size profiles establish store-level and national target distributions of sizes (S, M, L, etc.) at the product-color level for apparel items and are used to guide purchase and inventory movement decisions. Improved decision support that accounts for more than simply historical sales improves in-store and warehouse inventory balance, leading to reduced markdowns and stockouts and higher overall revenue-per-unit.
What questions is an inventory optimization project trying to answer?
The customer team threw a number of questions at Mosaic they wanted to answer including; what are the factors driving differences in demand across products/regions, at what level of the product hierarchy do size profiles vary, what is the impact of inventory imbalances on sales and margin/ what is the potential ROI from optimizing size profiles to better account for past inventory imbalances and stockouts.
Mosaic performed an extensive exploratory data analysis (EDA) to answer these questions and began to form the ideas of a custom data product to assist the retailer in their product placement decisions. The EDA explored and quantified patterns that emerged over time for particular sizes, stores, products, regions, customers, etc. Legacy demand forecasts were refreshed with machine learning techniques, allowing the merchandising team to more accurately capture demand, accounting for additional variables that drove purchasing behavior. Early EDA results gave Mosaic’s data scientists confidence in evaluating a number of different modeling approaches.
Machine Learning Evaluation & Custom Data Product Development
The customer and Mosaic decided on an automated predictive inventory planning tool that generated a recommended size profile for any product within the customer’s catalog. An ensemble model was designed using multiple algorithms to meet the retailers data inputs.
Mosaic designed the model with three key components
- Impute lost sales: Historical sales are adjusted to estimate lost sales during observed store-level stockouts.
- Product hierarchy aggregation: Products from past seasons are aggregated based on their brand, color, and place in the customer product hierarchy. If an insufficient volume of sales was observed within this group of products then multiple aggregation groups are combined.
- Size profile generation: Each product to be assorted is matched to the appropriate aggregation group. Based on the store assortment plan for the product and the historical observed and lost sales for the product’s aggregation group, a size profile is generated that recommends how an inventory buy for the product should be distributed across sizes.
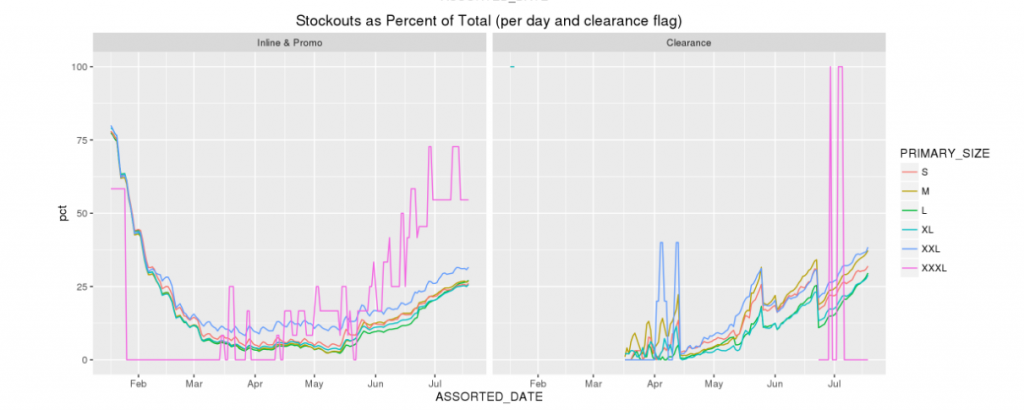
Saving Time & Money with Machine Learning
The retailer now uses this tool to plan not only for apparel and shoes, but for their entire product line. By embracing the power of data science, the client now better understands their product demand, allowing them to make decisions that decrease stockouts, reduce end-of-season markdowns, and increase revenues at the item level in an automated, custom data product.
Tying it all together
Meeting customer expectations is more difficult than ever, more and more of market share goes to companies who are able to perceive needs rather than react. Whether e-tailing or selling in brick-n-mortar stores, inventory planning is a promising area for predictive analytics.
Through the collection of biometrics, refreshed forecasts, customer clusters, and product features, executives can support their product placement decisions with lots of insights. Our data scientists are comfortable bringing merchandising teams from spreadsheet- or legacy software- approaches with substantial limitations, into a world where much more data can be utilized to make better decisions. This saves merchandising teams much needed time and allows them to place the right product at the right store at the right time.