In this Q&A, we sat with Principal Data Scientist Priya Karanth for her take on the latest innovations and most potent applications behind the ever-changing landscape of computer vision. Let’s see what lies beyond the surface.
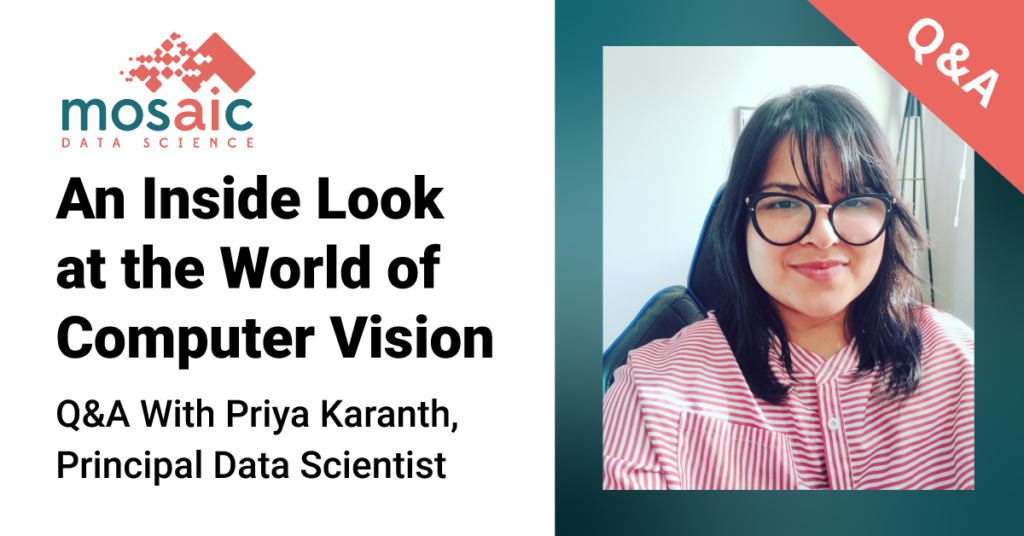
1. What are your official title, role, and responsibilities at Mosaic Data Science?
As a Principal Data Scientist at Mosaic, Priya Karanth helps clients build digital processes using machine learning techniques to guide a concept to a live solution. To Karanth, she does not just build machine learning models – it’s about ensuring that these analytics solutions bring value by ensuring safer processes, making customers’ lives easier, and helping them save on operations.
2. How did you first come across computer vision in your data science journey? What was your first project?
Karanth’s first computer vision project was in GE Global Research, where she was part of a research lab that built analytics for medical imaging devices in healthcare.
“There, I learned from the best and even today still follow the teachings and practices embedded early in my career,” says Karanth.
The project was to identify human livers in an ultrasound scan and build 3D projection models to assist in monitoring disease progression in patients and robotic surgeries.
Thus began Karanth’s productive and lengthy affair with computer vision.
3. What is computer vision? How does computer vision help companies make better use of their available data?
Karanth put it simply: computer vision is a field in data science that enables machines to see. Although not as capable as human vision, they can replace humans in situations where we need monitoring for extended periods of time.
There are many opportunities for companies in all domains to apply computer vision, even with the data they already possess. Karanth points to the possibilities surrounding monitoring, surveillance, and theft prevention of an organization’s office, operation, or retail facility. This can be achieved by extracting insights from existing camera feeds.
Another application and benefit of computer vision using camera feeds involves improving the efficiency of operations in warehouses. Karanth highlights the model’s ability to be trained to identify time-consuming tasks (such as scanning barcodes of packages delivered in bulk and ordering and stocking products in inventory tracking) or identifying defects of products in a production line.
These are just a few examples that open up a world of possibilities for computer vision when applied to an organization’s existing data.
4. What’s the coolest thing you have seen with a computer vision application?
“Computer vision in art might be my favorite thing I’ve seen,” says Karanth. “This process uses styleGANs and other novel techniques to generate paintings that borrow images and styles from various artists, combining them to create beautiful art pieces.”
StyleGAN (Generative Adversarial Network) is a revolutionary computer vision tool that has changed the image generation and style transfer fields forever. Its first version was released in 2018 and has continuously been updated for improvements.
StyleGAN has risen in popularity recently because of its astonishing results in generating natural-looking images. It could generate human faces, animals, cars, and landscapes. Using this tool, one can quickly generate interpolations between different images and make some changes to the image. For example, you can change the person’s mood in the picture, rotate the objects, etc.
5. What are the techniques and most promising use cases associated with this technology? Which industries can benefit?
“In my opinion, object detection segmentation and classification is the most basic technique in computer vision – but it can be applied to so many industries, making it highly valuable and useful,” says Karanth.
A few examples of applications of object detection can be found in the field of operations, where it is used to ensure PPE (personal protective equipment) is being worn by all personnel. Other examples include disease identification in healthcare, animal monitoring in smart farming, crop farming in precision agriculture, and monitoring traffic violations.
6. How do you think computer vision ties into other deep learning/ML applications?
“Computer vision has some overlap with machine learning/deep learning and robotics,” explains Karanth. “By helping computers understand and interpret the visual world, it can be used for descriptive analytics to understand operations, efficiency, and to make better decisions. In this process, a feedback loop is created suggesting action to take from the information deduced.”
Karanth shares an example of a parking lot monitoring application scenario, where a vision feed could help measure the efficiency of said lot. Through video, a computer vision model can determine the number of times the lot was fully utilized and if the spacing of the parking lines created is adequate enough to avoid vehicle collisions or monitor for any vehicles parked beyond the allotted time frame. Depending on the results of such analyses, a business can determine the next course of action.
7. Why do you think computer vision problems are so significant to solve? What are the benefits and limitations of computer vision?
“Using computer vision is an advancement to the future,” says Karanth. “This technology makes the impossible possible. Who would have thought cars could be driven autonomously? We only saw this in movies growing up. Today, you can walk out of a grocery store by picking an item from a shelf with no scanning, no cashiers, no cards – and computer vision alongside additional analytics made it possible.”
On the flip side, there are some limitations Karanth wants us all to keep in mind as we continue to develop computer vision technology: speed.
“Although computers are fast, they are still not as fast as we need them to be on edge devices,” Karanth says. “There are limitations on the kind of algorithms that are precise enough to be applied on edge devices. Many are not accurate enough to run completely without human supervision.”
Finally, Karanth has found that labeling the data has proven to be another challenge for training computer vision models; however, the trend is shifting from big data to quality data, which means there is a general industry focus on iteratively improving the quality of labeling.
“Despite its growing pains, computer vision has something to offer to improve every industry and human life – which is why it is so important to solve these problems,” Karanth says. “It’s also why I’m proud to work alongside my team at Mosaic Data Science to innovate and help our customers with their biggest challenges.”