Summary
Mosaic deployed unsupervised learning to customer segmentation for a leading retail energy company.
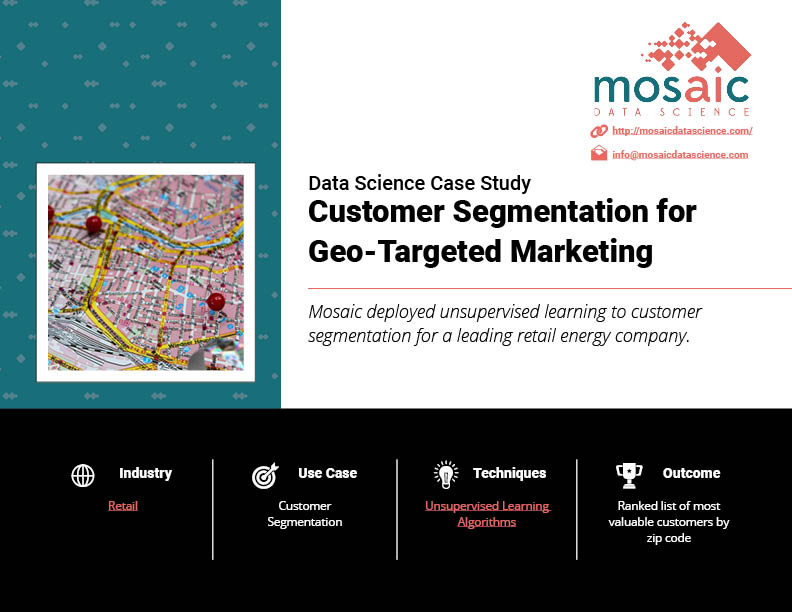
Take Our Content to Go
Overview
In today’s hypercompetitive business environment, marketing teams need to maximize the return on every dollar spent. This means having a clear idea of what type of customers one wants to attract, how to find them, and what messages to send to turn prospects into purchasers. The modern marketer is expected to get the most out of their budgets, growing the top line through new customer acquisition while keeping expenses down and boosting the bottom line through effective advertising. Business stakeholders expect marketing departments to know their customer inside and out. Predictive analytics can bring critical improvements to this process, increasing the efficiency of marketing efforts through segmentation and geo-targeting.
A national residential energy supplier approached Mosaic, a leading machine learning consulting firm, because they wanted to understand current customers and to identify high potential areas to target for new customer acquisition. Specifically, they wanted to make sure to direct outreach campaigns and online ad spending towards the precise locations most likely to contain high-value prospects.
Mosaic fused internal and external data from the organization and used sophisticated unsupervised machine learning algorithms to segment current customers and identify high-growth geographic regions.
Unsupervised Customer Segmentation
Mosaic first met with business stakeholders to determine what internal customer data was available and to generate hypotheses about which types of information might relate to long-term customer value. After these initial discussions, the data science consultant team extracted more than 20 different types of transaction details – from volume of energy purchased to frequency of customer service calls. The team then used powerful dimensionality reduction techniques to combine these 20+ features into two over-arching dimensions: brand loyalty and energy volume. After that, an unsupervised machine learning algorithm was used to segment residential customers based on the loyalty and energy volume dimensions, because customers that are both using high volumes and are loyal to the brand are likely to have high long-term customer value.
Once customers were separated into eight groups, or clusters, the goal was to identify the highest-value customer types and create demographic profiles of the areas in which those customers lived. The idea was that if high-value customers tended to live in certain areas (e.g., regions with above-average income), then it was possible that new customers from similar regions would also be valuable to the company. The Mosaic team did this by evaluating which segments were generating a higher proportion of revenue than their share of the customer base, as shown in Figure 1. It’s clear from the image that customers in clusters 5, 6 and 8 – representing just over a quarter of the company’s residential customers – were generating almost 60% of revenue. In contrast, clusters 3 and 5 contained a similar number of customers but only contributed 5% to overall revenue. Thus, the company could stand to gain a lot by focusing lead generation efforts on households similar to those in the three high-value segments.
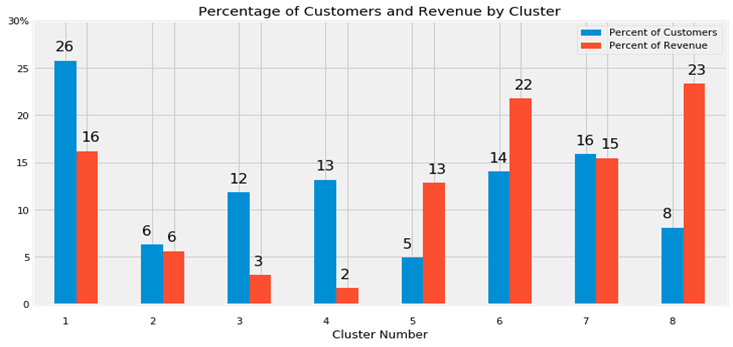
The machine learning consultants then mapped residential customers to external public data from the U.S. Census and American Community Survey based on the zip codes in which customers in the high-value clusters lived. A combination of six demographic features differentiated customers in these three groups from all other customers, and from the U.S. as a whole. As a result, Mosaic’s data scientists rated all U.S. zip codes based on how closely they matched high-value customers’ locations on these six demographic features. Zip codes that matched at least one cluster on all six features were identified as potential matches for targeted customer acquisition.
The question remained, though, of the size of the potential market in each location, as the ROI on marketing would depend on the number of possible new customers in addition to those customers’ profitability. Mosaic therefore further screened the zip codes based on their total populations and primary sources of home heating to estimate the total market in each region. Zip codes with at least 100 potential customers remained in the list of high-opportunity locations.
Unsupervised Customer Segmentation Results
Mosaic identified 1,639 U.S. zip codes likely to contain high-value prospects for the energy company, as shown in the map in Figure 2. Some of these areas are very close to the company’s existing service territories, and are therefore going to be targeted in upcoming marketing campaigns. Others are farther from current service areas, and these zip codes will be used to evaluate potential M&A opportunities, as the company looks to expand through acquisition to new territories.
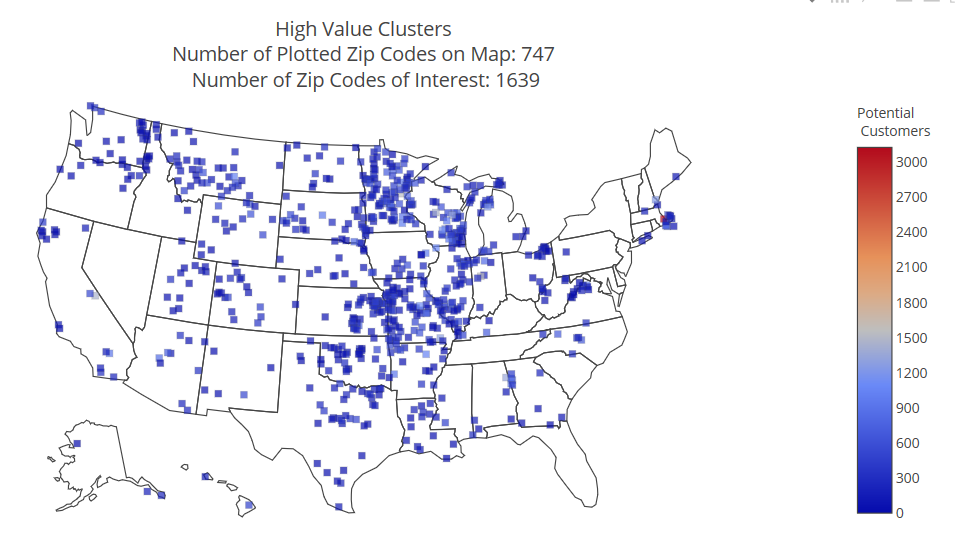
Through this unsupervised customer segmentation project, Mosaic’s client learned about their customers’ buying behaviors and which types of customers brought in the most value. In addition, the marketing team learned where their greatest opportunities exist. This means that marketing dollars will be spent more efficiently, targeting areas not just with a large number of prospects, but specifically areas with a large number of highly profitable customers.
0 Comments